GA-CCRi’s conference speaking stars
Here at GA-CCRi, we’re working on such an interesting set of technologies that we could probably find a conference to speak at every week if we wanted to. Two especially nice conferences that recently included GA-CCRi speakers were the Google Cloud Next ‘17 conference and Charlottesville’s Tom Tom Festival Machine Learning conference.
As described by Forbes magazine, Google Cloud Next is “Google’s chance to showcase its achievements from the past year, as well as announce new products and services within their cloud and collaboration portfolio.” Over 10,000 attendees chose from over 200 sessions at this year’s three-day conference in San Francisco’s Moscone Center.
One session included GA-CCRi’s Director of Data Science and System Architecture Anthony Fox, who spoke about using open source technology (in particular, GeoMesa) to manage spatio-temporal data with Google Cloud Bigtable, Google’s massively scalable NoSQL database.
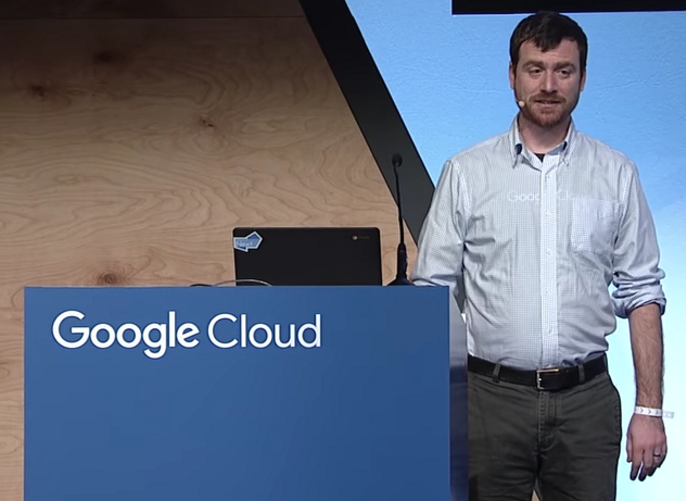
Anthony described how GeoMesa uses space-filling curves to take the most efficient advantage of Google Cloud Bigtable’s native indexing features and Bigtable’s Spark support to provide efficient analytics. This enabled the answering of questions such as “What is the optimal time of day to traverse the Panama canal to avoid traffic?” His entire talk is available on YouTube.
A speaking engagement last week was much closer to home. GA-CCRi is located in Charlottesville, Virginia, which is also home to the week-long celebration of innovation, art, music, and food known as the Tom Tom Founders Festival. (Like many things in Charlottesville, the festival is named for Thomas Jefferson, who built Monticello and founded the University of Virginia here.) This year’s sixth annual festival included a half-day machine learning conference where GA-CCRi’s Taryn Price and Courtney Shindeldecker gave a keynote presentation titled “Learn about Your Location (Using ALL Your Data).”

Over 170 people sat in the plush seats of the Violet Crown movie theater to hear Taryn describe how geospatial data, text, and satellite imagery can connect together in a graph structure once they are converted to a representation known as triples. These triples can then be converted into standard numeric vectors known as embeddings to use for modeling. As an example, Taryn showed how the fused information lets us find out more about different parts of Chicago represented on a color-coded map. Courtney went on to describe how algorithms can learn these vectors and then leverage them to enable classification, similarity search, and clustering of the represented entities so that we can learn more about the sections of a geographic region (in the slide shown above, New Orleans and the shipping lanes of the Gulf of Mexico) in ways that can benefit everything from ecology research to agriculture and retail planning.
You can see Taryn and Courtney’s slides on GA-CCRi’s slideshare page. Slideshare had difficulty showing their animation of how, when an algorithm learns the vectors in an embedding space, it eventually groups similar entities together, but you can see it right here:

We’re looking forward to next year’s Tom Tom Fest Machine learning festival!